What Is Informatics?
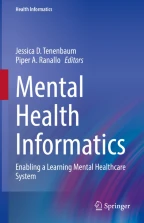
Biomedical informatics is a discipline dating back to the 1950s that continues to evolve with the growth of data and advances in technology in biomedicine and health care. This field brings together foundational approaches from many scientific and technological disciplines that can be applied across the spectrum from molecules to individuals to populations. In the context of the learning healthcare system, this chapter highlights frameworks and methods for transforming data to knowledge, putting knowledge into practice through evidence-based technology innovations, and evaluating the impact of those innovations.
This is a preview of subscription content, log in via an institution to check access.
Access this chapter
Subscribe and save
Springer+ Basic
€32.70 /Month
- Get 10 units per month
- Download Article/Chapter or eBook
- 1 Unit = 1 Article or 1 Chapter
- Cancel anytime
Buy Now
Price includes VAT (France)
eBook EUR 50.28 Price includes VAT (France)
Softcover Book EUR 63.29 Price includes VAT (France)
Hardcover Book EUR 89.66 Price includes VAT (France)
Tax calculation will be finalised at checkout
Purchases are for personal use only
Similar content being viewed by others
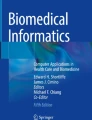
Biomedical Informatics: The Science and the Pragmatics
Chapter © 2021
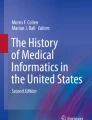
The Creation of a New Discipline
Chapter © 2015
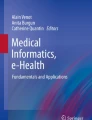
Medical Informatics as a Scientific Discipline
Chapter © 2014
References
- Collen MF. A history of medical informatics in the United States, 1950 to 1990, vol. xv. Indianapolis, IN: American Medical Informatics Association; 1995. p. 489. Google Scholar
- J Ball M. The history of medical informatics in the United States. New York, NY: Springer Berlin Heidelberg; 2015. Google Scholar
- Greenes RA, Shortliffe EH. Medical informatics. An emerging academic discipline and institutional priority. JAMA. 1990;263(8):1114–20. CASPubMedGoogle Scholar
- Hagen JB. The origins of bioinformatics. Nat Rev Genet. 2000;1(3):231–6. CASPubMedGoogle Scholar
- Martin-Sanchez F, et al. Synergy between medical informatics and bioinformatics: facilitating genomic medicine for future health care. J Biomed Inform. 2004;37(1):30–42. CASPubMedGoogle Scholar
- Maojo V, et al. Medical informatics and bioinformatics: European efforts to facilitate synergy. J Biomed Inform. 2001;34(6):423–7. CASPubMedGoogle Scholar
- Kulikowski CA, et al. AMIA board white paper: definition of biomedical informatics and specification of core competencies for graduate education in the discipline. J Am Med Inform Assoc. 2012;19(6):931–8. PubMedPubMed CentralGoogle Scholar
- Oxford English Dictionary, O.E., “informatics, n.”. Oxford University Press. Google Scholar
- Oxford English Dictionary, O.E., “bioinformatics, n.”. Oxford University Press. Google Scholar
- Luscombe NM, Greenbaum D, Gerstein M. What is bioinformatics? A proposed definition and overview of the field. Methods Inf Med. 2001;40(4):346–58. CASPubMedGoogle Scholar
- van Bemmel JH. The structure of medical informatics. Med Inform (Lond). 1984;9(3–4):175–80. Google Scholar
- Bemmel JHv, Musen MA, Helder JC. Handbook of medical informatics, vol. xl. AW Houten, Netherlands, Heidelberg, Germany: Bohn Stafleu Van Loghum: Springer Verlag; 1997. 621 p. Google Scholar
- Shortliffe E, Cimino J. Biomedical informatics, vol. 12. London: Springer London; 2014. https://doi.org/10.1007/978-1-4471-4474-8. BookGoogle Scholar
- Bernstam EV, Smith JW, Johnson TR. What is biomedical informatics? J Biomed Inform. 2010;43(1):104–10. PubMedGoogle Scholar
- Valenta AL, et al. AMIA board white paper: AMIA 2017 core competencies for applied health informatics education at the master’s degree level. J Am Med Inform Assoc. 2018;25(12):1657–68. PubMedPubMed CentralGoogle Scholar
- Mantas J, et al. Recommendations of the international medical informatics association (IMIA) on education in biomedical and health informatics. First revision. Methods Inf Med. 2010;49(2):105–20. PubMedGoogle Scholar
- Jaspers MW, et al. IMIA accreditation of biomedical and health informatics education: current state and future directions. Yearb Med Inform. 2017;26(1):252–6. CASPubMedPubMed CentralGoogle Scholar
- Chen ES, Sarkar IN. Informatics: identifying and tracking informatics sub-discipline terms in the literature. Methods Inf Med. 2015;54(6):530–9. CASPubMedGoogle Scholar
- Hersh W. A stimulus to define informatics and health information technology. BMC Med Inform Decis Mak. 2009;9:24. PubMedPubMed CentralGoogle Scholar
- Kuhn KA, et al. Informatics and medicine–from molecules to populations. Methods Inf Med. 2008;47(4):283–95. CASPubMedGoogle Scholar
- Payne PR, Embi PJ, Sen CK. Translational informatics: enabling high-throughput research paradigms. Physiol Genomics. 2009;39(3):131–40. Google Scholar
- Payne PRO, Bernstam EV, Starren JB. Biomedical informatics meets data science: current state and future directions for interaction. JAMIA Open. 2018;1(2):136–141. Google Scholar
- Boland MR, et al. Birth month affects lifetime disease risk: a phenome-wide method. J Am Med Inform Assoc. 2015;22(5):1042–53. PubMedPubMed CentralGoogle Scholar
- Sarkar IN. Biomedical informatics and translational medicine. J Transl Med. 2010;8:22. PubMedPubMed CentralGoogle Scholar
- Ball MJ, Hannah KJ. Nursing informatics: where technology and caring meet, Health informatics, vol. xxxi. 4th ed. London; New York: Springer; 2011. p. 482. Google Scholar
- Saba VK, McCormick KA. Essentials of nursing informatics, vol. xxiii. 6th ed. New York: McGraw-Hill Education; 2015. p. 886. Google Scholar
- Abbey LM, Zimmerman JL. Dental informatics: integrating technology into the dental environment. Computers in health care, vol. xiii. New York: Springer; 1992. p. 348. Google Scholar
- Lewis D. Consumer health informatics: informing consumers and improving health care. Health informatics. New York: Springer; 2005. p. xxi, 258 p. Google Scholar
- Consumer informatics and digital health: solutions for health and health care. 2018, New York, NY: Springer Science+Business Media, LLC. pages cm. Google Scholar
- Payne PR, Embi PJ. Translational informatics: realizing the promise of knowledge-driven healthcare: Springer; 2014. Google Scholar
- Butte AJ. Translational bioinformatics: coming of age. J Am Med Inform Assoc. 2008;15(6):709–14. PubMedPubMed CentralGoogle Scholar
- Sarkar IN, et al. Translational bioinformatics: linking knowledge across biological and clinical realms. J Am Med Inform Assoc. 2011;18(4):354–7. PubMedPubMed CentralGoogle Scholar
- Tenenbaum JD. Translational bioinformatics: past, present, and future. Genomics Proteomics Bioinformatics. 2016;14(1):31–41. PubMedPubMed CentralGoogle Scholar
- Richesson RL, Andrews JE. Clinical research informatics. Health informatics, vol. ix. London; New York: Springer; 2012. 419 pages. Google Scholar
- Dixon BE, et al. What's past is prologue: a scoping review of recent public health and Global Health informatics literature. Online J Public Health Inform. 2015;7(2):e216. PubMedPubMed CentralGoogle Scholar
- Silva JS. Cancer informatics: essential technologies for clinical trials. Health informatics, vol. xxvi. New York: Springer; 2002. p. 377. Google Scholar
- Oli AN, et al. Immunoinformatics and vaccine development: an overview. Immunotargets Ther. 2020;9:13–30. CASPubMedPubMed CentralGoogle Scholar
- Hanson A, Levin BL. Mental health informatics, vol. x. New York: Oxford University Press; 2013. p. 274. Google Scholar
- Diederich J, Song I. Mental health informatics: current approaches, in Mental Health Informatics: Springer; 2014. p. 1–16. Google Scholar
- Lehmann CU, Kim GR, Johnson KB. Pediatric informatics: computer applications in child health. Health informatics series, vol. xxiv. Dordrecht; New York: Springer; 2009. 483 p. Google Scholar
- Pantanowitz L, et al. Pathology informatics: theory & practice, vol. xvi. Chicago, Ill: American Society for Clinical Pathology Press; 2012. 352 p. Google Scholar
- Sinard JH. Practical pathology informatics: demystifying informatics for the practicing anatomic pathologist, vol. xix. New York: Springer Science+Business Media; 2006. p. 393. Google Scholar
- Lustgarten J. et al. Veterinary informatics: forging the future between veterinary medicine, human medicine, and One Health initiatives—a joint paper by the Association of Veterinary Informatics (AVI) and the CTSA One Health Alliance (COHA). JAMIA Open, 2020. Google Scholar
- Institute of Medicine (U.S.). Roundtable on Value & Science-Driven Health Care, et al. Digital infrastructure for the learning health system: the foundation for continuous improvement in health and health care: workshop series summary. Learning health system series, vol. xxvi. Washington, D.C: National Academies Press; 2011. 308 p. Google Scholar
- Friedman C, et al. Toward a science of learning systems: a research agenda for the high-functioning learning health system. J Am Med Inform Assoc. 2015;22(1):43–50. PubMedGoogle Scholar
- Friedman CP, Rubin JC, Sullivan KJ. Toward an information infrastructure for Global Health improvement. Yearb Med Inform. 2017;26(1):16–23. CASPubMedPubMed CentralGoogle Scholar
- Flynn AJ, et al. The knowledge object reference ontology (KORO): a formalism to support management and sharing of computable biomedical knowledge for learning health systems. Learn Health Syst. 2018;2(2):e10054. PubMedPubMed CentralGoogle Scholar
- Sarkar IN. Methods in biomedical informatics: a pragmatic approach. Amsterdam: Elsevier/AP, Academic Press is an imprint of Elsevier; 2014. p. xvi, 571 pages. Google Scholar
- Ackoff RL. From data to wisdom. J Appl Syst Anal. 1989;16(1):3–9. Google Scholar
- Zins C. Conceptual approaches for defining data, information, and knowledge. J Am Soc Info Sci Tech. 2007;58(4):479–93. Google Scholar
- Rowley J. The wisdom hierarchy: representations of the DIKW hierarchy. J Inf Sci. 2007;33(2):163–80. Google Scholar
- Matney S, et al. Philosophical approaches to the nursing informatics data-information-knowledge-wisdom framework. ANS Adv Nurs Sci. 2011;34(1):6–18. PubMedGoogle Scholar
- Ronquillo C, Currie LM, Rodney P. The evolution of data-information-knowledge-wisdom in nursing informatics. ANS Adv Nurs Sci. 2016;39(1):E1–18. PubMedGoogle Scholar
- Dammann O. Data, information, evidence, and knowledge:: a proposal for health informatics and data science. Online J Public Health Inform. 2018;10(3):e224. PubMedGoogle Scholar
- Piateski G, Frawley W. Knowledge discovery in databases. 1991: MIT press. Google Scholar
- Fayyad U, Piatetsky-Shapiro G, Smyth P. From data mining to knowledge discovery in databases. AI Mag. 1996;17(3):37. Google Scholar
- Kurgan LA, Musilek P. A survey of knowledge discovery and data mining process models. Knowl Eng Rev. 2006;21(1):1–24. Google Scholar
- Mason H, Wiigins C. A taxonomy of data science. Available from: http://www.dataists.com/2010/09/a-taxonomy-of-data-science/.
- Janssens J, editor. Data science at the command line, vol. xvii. 1st ed. Sebastopol, CA: O’Reilly; 2014. 191 pages. Google Scholar
- Yin S, Kaynak O. Big data for modern industry: challenges and trends [point of view]. Proc IEEE. 2015;103(2):143–6. Google Scholar
- Jagadish H. Big data and science: myths and reality. Big Data Res. 2015;2(2):49–52. Google Scholar
- Berman JJ. Principles of big data: preparing, sharing, and analyzing complex information, vol. xxvi. Amsterdam: Elsevier, Morgan Kaufmann; 2013. 261 pages. Google Scholar
- Zikopoulos P. Understanding big data: analytics for enterprise class Hadoop and streaming data, vol. xxxi. New York: McGraw-Hill; 2012. 141 pages Google Scholar
- Weber GM, Mandl KD, Kohane IS. Finding the missing link for big biomedical data. JAMA. 2014;311(24):2479–80. CASPubMedGoogle Scholar
- Tan SS, Gao G, Koch S. Big data and analytics in healthcare. Methods Inf Med. 2015;54(6):546–7. PubMedGoogle Scholar
- Ross MK, Wei W, Ohno-Machado L. “Big data” and the electronic health record. Yearb Med Inform. 2014;9:97–104. CASPubMedPubMed CentralGoogle Scholar
- Chen ES, Sarkar IN. Mining the electronic health record for disease knowledge. Methods Mol Biol. 2014;1159:269–86. PubMedGoogle Scholar
- Gandomi A, Haider M. Beyond the hype: big data concepts, methods, and analytics. Int J Inf Manag. 2015;35(2):137–44. Google Scholar
- Collen MF. Computer medical databases: the first six decades (1950–2010). Health informatics, vol. xix. London; New York: Springer; 2012. 288 p. Google Scholar
- Beam AL, Kohane IS. Big data and machine learning in health care. JAMA. 2018;319(13):1317–8. PubMedGoogle Scholar
- Riley J. Understanding metadata. National Information Standards Organization, 2017. Google Scholar
- Zhang AB, Gourley D. Creating digital collections: a practical guide. Chandos information professional series. 2009, Oxford: Chandos Pub xiv, 234 p. Google Scholar
- Wilkinson MD, et al. The FAIR guiding principles for scientific data management and stewardship. Sci Data. 2016;3:160018. PubMedPubMed CentralGoogle Scholar
- Ramakrishnan R, Gehrke J. Database management systems, vol. xxxii. 3rd ed. Boston: McGraw-Hill; 2003. p. 1065. Google Scholar
- Codd EF. The relational model for database management: version 2, vol. xxii. Reading, Mass: Addison-Wesley; 1990. 538 p Google Scholar
- Jatana N, et al. A survey and comparison of relational and non-relational database. Int J Eng Res Tech. 2012;1(6):1–5. Google Scholar
- Sholle ET, et al. Secondary use of Patients' electronic records (SUPER): an approach for meeting specific data needs of clinical and translational researchers. AMIA Annu Symp Proc. 2017;2017:1581–8. PubMedGoogle Scholar
- Stein B, Morrison A. The enterprise data lake: Better integration and deeper analytics. Google Scholar
- Gorelik A. The enterprise big data lake: delivering the promise of big data and data science, vol. xiii. 1st ed. Sebastopol, California: iO’Reilly Media, Inc; 2019. 205 pages. Google Scholar
- Ford E, et al. Extracting information from the text of electronic medical records to improve case detection: a systematic review. J Am Med Inform Assoc. 2016;23(5):1007–15. PubMedPubMed CentralGoogle Scholar
- Richesson RL, et al. Electronic health records based phenotyping in next-generation clinical trials: a perspective from the NIH health care systems Collaboratory. J Am Med Inform Assoc. 2013;20(e2):e226–31. PubMedPubMed CentralGoogle Scholar
- Hripcsak G, Albers DJ. Next-generation phenotyping of electronic health records. J Am Med Inform Assoc. 2013;20(1):117–21. PubMedGoogle Scholar
- Shivade C, et al. A review of approaches to identifying patient phenotype cohorts using electronic health records. J Am Med Inform Assoc. 2014;21(2):221–30. PubMedGoogle Scholar
- Wei WQ, Denny JC. Extracting research-quality phenotypes from electronic health records to support precision medicine. Genome Med. 2015;7(1):41. PubMedPubMed CentralGoogle Scholar
- Richesson RL, et al. Clinical phenotyping in selected national networks: demonstrating the need for high-throughput, portable, and computational methods. Artif Intell Med. 2016;71:57–61. PubMedPubMed CentralGoogle Scholar
- Pendergrass SA, Crawford DC. Using electronic health records to generate phenotypes for research. Curr Protoc Hum Genet. 2019;100(1):e80. PubMedGoogle Scholar
- Harris PA, et al. Research electronic data capture (REDCap)–a metadata-driven methodology and workflow process for providing translational research informatics support. J Biomed Inform. 2009;42(2):377–81. PubMedGoogle Scholar
- Devine EB, et al. Health information exchange use (1990-2015): a systematic review. EGEMS (Wash DC). 2017;5(1):27. Google Scholar
- Menachemi N, et al. The benefits of health information exchange: an updated systematic review. J Am Med Inform Assoc. 2018;25(9):1259–65. PubMedPubMed CentralGoogle Scholar
- Peters A, et al. The value of all-payer claims databases to states. N C Med J. 2014;75(3):211–3. PubMedGoogle Scholar
- Electronic Health Records-Based Phenotyping. Available from: https://rethinkingclinicaltrials.org/resources/ehr-phenotyping/.
- Jurafsky D, Martin JH. Speech and language processing: an introduction to natural language processing, computational linguistics, and speech recognition, Prentice Hall series in artificial intelligence, vol. xxxi. 2nd ed. Upper Saddle River, N.J: Pearson Prentice Hall; 2009. 988 p. Google Scholar
- Allen JF. Natural language processing. 2003. Google Scholar
- Filannino M, Uzuner O. Advancing the state of the art in clinical natural language processing through shared tasks. Yearb Med Inform. 2018;27(1):184–92. PubMedPubMed CentralGoogle Scholar
- Velupillai S, et al. Using clinical natural language processing for health outcomes research: overview and actionable suggestions for future advances. J Biomed Inform. 2018;88:11–9. PubMedPubMed CentralGoogle Scholar
- Cohen KB, Demner-Fushman D. Biomedical natural language processing. Natural language processing (NLP), vol. x. Amsterdam; Philadelphia: John Benjamins Publishing Company; 2014. 160 pages. Google Scholar
- Doan S, et al. Natural language processing in biomedicine: a unified system architecture overview. Methods Mol Biol. 2014;1168:275–94. PubMedGoogle Scholar
- Conway M, Hu M, Chapman WW. Recent advances in using natural language processing to address public Health Research questions using social media and ConsumerGenerated data. Yearb Med Inform. 2019;28(1):208–17. PubMedPubMed CentralGoogle Scholar
- Hearst MA. Untangling text data mining. In Proceedings of the 37th annual meeting of the Association for Computational Linguistics on Computational Linguistics. 1999. Association for Computational Linguistics. Google Scholar
- Zweigenbaum P, et al. Frontiers of biomedical text mining: current progress. Brief Bioinform. 2007;8(5):358–75. CASPubMedGoogle Scholar
- Shatkay H, Feldman R. Mining the biomedical literature in the genomic era: an overview. J Comput Biol. 2003;10(6):821–55. CASPubMedGoogle Scholar
- Kumar VD, Tipney HJ. Biomedical literature mining. Methods in molecular biology, vol. xii. New York: Humana Press; 2014. 288 p. Google Scholar
- Nadkarni PM, Ohno-Machado L, Chapman WW. Natural language processing: an introduction. J Am Med Inform Assoc. 2011;18(5):544–51. PubMedPubMed CentralGoogle Scholar
- Wang Y, et al. Clinical information extraction applications: a literature review. J Biomed Inform. 2018;77:34–49. PubMedGoogle Scholar
- Available from: http://medicaltranscriptionwordhelp.synthasite.com/mental-status-exam-common-words-and-phrases.php.
- Hedley D, et al. Risk and protective factors underlying depression and suicidal ideation in autism Spectrum disorder. Depress Anxiety. 2018;35(7):648–57. PubMedGoogle Scholar
- Sentiment140 dataset with 1.6 million tweets. Available from: https://www.kaggle.com/kazanova/sentiment140.
- Russell SJ, Norvig P. Artificial intelligence: a modern approach. Fourth edition. Ed. Pearson series in artificial intelligence. Hoboken: Pearson. pages cm; 2021. Google Scholar
- Shalev-Shwartz S, Ben-David S. Understanding machine learning: from theory to algorithms, vol. xvi. New York, NY, USA: Cambridge University Press; 2014. 397 pages. Google Scholar
- Bishop CM. Pattern recognition and machine learning. Information science and statistics. New York: Springer; 2006. p. xx, 738 p. Google Scholar
- Yu KH, Beam AL, Kohane IS. Artificial intelligence in healthcare. Nat Biomed Eng. 2018;2(10):719–31. PubMedGoogle Scholar
- Witten IH, Witten IH. Data mining: practical machine learning tools and techniques, vol. xxxii. 4th ed. Amsterdam: Elsevier; 2017. p. 621. Google Scholar
- Maini V, Sabri S, Machine learning for humans. Online: https://medium.com/machine-learning-for-humans, 2017.
- Rajkomar A, Dean J, Kohane I. Machine learning in medicine. N Engl J Med. 2019;380(14):1347–58. PubMedGoogle Scholar
- Wang F, Casalino LP, Khullar D. Deep learning in medicine-promise, Progress, and challenges. JAMA Intern Med. 2019;179(3):293–4. PubMedGoogle Scholar
- Esteva A, et al. A guide to deep learning in healthcare. Nat Med. 2019;25(1):24–9. CASPubMedGoogle Scholar
- Chen ES, Melton GB, Sarkar IN. Translating standards into practice: experiences and lessons learned in biomedicine and health care. J Biomed Inform. 2012;45(4):609–12. PubMedGoogle Scholar
- Dolin RH, Alschuler L. Approaching semantic interoperability in health level seven. J Am Med Inform Assoc. 2011;18(1):99–103. PubMedGoogle Scholar
- Overhage JM, et al. Validation of a common data model for active safety surveillance research. J Am Med Inform Assoc. 2012;19(1):54–60. PubMedGoogle Scholar
- Murphy SN, et al. Serving the enterprise and beyond with informatics for integrating biology and the bedside (i2b2). J Am Med Inform Assoc. 2010;17(2):124–30. PubMedPubMed CentralGoogle Scholar
- Klann JG, et al. Data model harmonization for the all of us research program: transforming i2b2 data into the OMOP common data model. PLoS One. 2019;14(2):e0212463. CASPubMedPubMed CentralGoogle Scholar
- Schulz S, Stegwee R, Chronaki C. Standards in healthcare data, in fundamentals of clinical data science, P. Kubben, M. Dumontier, and A. Dekker, Editors. 2019: Cham (CH). p. 19–36. Google Scholar
- Hammond WE. The making and adoption of health data standards. Health Aff (Millwood). 2005;24(5):1205–13. Google Scholar
- About HL7. Available from: https://www.hl7.org/about/index.cfm?ref=nav.
- Benson T. Principles of health interoperability: snomed ct, hl7 and fhir. New York, NY: Springer Berlin Heidelberg. pages cm; 2016. Google Scholar
- HL7 Implementation Guides. Available from: https://www.hl7.org/implement/standards/product_section.cfm?section=22&ref=nav.
- Interoperability Standards Advisory. Available from: https://www.healthit.gov/isa/.
- Cimino JJ. Review paper: coding systems in health care. Methods Inf Med. 1996;35(4–5):273–84. CASPubMedGoogle Scholar
- Cimino JJ, Zhu X. The practical impact of ontologies on biomedical informatics. Yearb Med Inform. 2006:124–35. Google Scholar
- U.S. Core Data for Interoperability (USCDI). Available from: https://www.healthit.gov/isa/us-core-data-interoperability-uscdi.
- Bodenreider O, Cornet R, Vreeman DJ. Recent developments in clinical terminologies - SNOMED CT, LOINC, and RxNorm. Yearb Med Inform. 2018;27(1):129–39. PubMedPubMed CentralGoogle Scholar
- Lindberg C. The unified medical language system (UMLS) of the National Library of medicine. J Am Med Rec Assoc. 1990;61(5):40–2. CASPubMedGoogle Scholar
- McCray AT, Nelson SJ. The representation of meaning in the UMLS. Methods Inf Med. 1995;34(1–2):193–201. CASPubMedGoogle Scholar
- Noy NF, et al. BioPortal: ontologies and integrated data resources at the click of a mouse. Nucleic Acids Res. 2009;37(Web Server issue):W170–3. CASPubMedPubMed CentralGoogle Scholar
- Musen MA, et al. The National Center for biomedical ontology. J Am Med Inform Assoc. 2012;19(2):190–5. PubMedGoogle Scholar
- McCray AT, Burgun A, Bodenreider O. Aggregating UMLS semantic types for reducing conceptual complexity. Stud Health Technol Inform. 2001;84(Pt 1):216–20. CASPubMedPubMed CentralGoogle Scholar
- Salsabili M, Kiogou S, Adam TJ. The evaluation of clinical classifications software using the National Inpatient Sample Database. AMIA Jt Summits Transl Sci Proc. 2020;2020:542–51. PubMedPubMed CentralGoogle Scholar
- Wu P, et al. Mapping ICD-10 and ICD-10-CM codes to Phecodes: workflow development and initial evaluation. JMIR Med Inform. 2019;7(4):e14325. PubMedPubMed CentralGoogle Scholar
- Wei WQ, et al. Evaluating phecodes, clinical classification software, and ICD-9-CM codes for phenome-wide association studies in the electronic health record. PLoS One. 2017;12(7):e0175508. PubMedPubMed CentralGoogle Scholar
- Digital health. Scaling healthcare to the world. New York, NY: Springer Berlin Heidelberg. pages cm; 2017. Google Scholar
- Digital Health. Available from: https://www.fda.gov/medical-devices/digital-health.
- World Health Organization, W.H., Recommendations on Digital Interventions for Health System Strengthening. 2019. Google Scholar
- Osheroff JA, et al. A roadmap for national action on clinical decision support. J Am Med Inform Assoc. 2007;14(2):141–5. PubMedPubMed CentralGoogle Scholar
- Greenes RA. Clinical decision support: the road to broad adoption, vol. xxxix. 2nd ed. Amsterdam Boston: Academic; 2014. p. 887. Google Scholar
- Tcheng JE, National Academy of Medicine (U.S.). Optimizing strategies for clinical decision support: summary of a meeting series, in The learning health system series. Washington, DC: National Academy of Medicine; 2017. p. 1 online resource Google Scholar
- Osheroff JA, Healthcare Information and Management Systems Society. Improving outcomes with clinical decision support: an implementer's guide, vol. xxiii. 2nd ed. Chicago, IL: HIMSS; 2012. p. 323. Google Scholar
- Bell GC, et al. Development and use of active clinical decision support for preemptive pharmacogenomics. J Am Med Inform Assoc. 2014;21(e1):e93–9. PubMedGoogle Scholar
- Cook DA, et al. Context-sensitive decision support (infobuttons) in electronic health records: a systematic review. J Am Med Inform Assoc. 2017;24(2):460–8. PubMedGoogle Scholar
- Introduction to Software Engineering/Process/Life Cycle. Available from: https://en.wikibooks.org/wiki/Introduction_to_Software_Engineering/Process/Life_Cycle.
- Kushniruk A. Evaluation in the design of health information systems: application of approaches emerging from usability engineering. Comput Biol Med. 2002;32(3):141–9. PubMedGoogle Scholar
- Sommerville I. Software engineering, vol. xv. 9th ed. Boston: Pearson; 2011. p. 773. Google Scholar
- Luna D, et al. User-centered design to develop clinical applications. Literature review. Stud Health Technol Inform. 2015;216:967. PubMedGoogle Scholar
- Kushniruk A, Nohr C. Participatory design, user involvement and health IT evaluation. Stud Health Technol Inform. 2016;222:139–51. PubMedGoogle Scholar
- Payne PR. Chapter 1: Biomedical knowledge integration. PLoS Comput Biol. 2012;8(12):e1002826. CASPubMedPubMed CentralGoogle Scholar
- Shortliffe EH, et al. Computer-based consultations in clinical therapeutics: explanation and rule acquisition capabilities of the MYCIN system. Comput Biomed Res. 1975;8(4):303–20. CASPubMedGoogle Scholar
- Pryor TA, Hripcsak G. The Arden syntax for medical logic modules. Int J Clin Monit Comput. 1993;10(4):215–24. CASPubMedGoogle Scholar
- Hripcsak G. Writing Arden syntax medical logic modules. Comput Biol Med. 1994;24(5):331–63. CASPubMedGoogle Scholar
- Wright A, Sittig DF. A four-phase model of the evolution of clinical decision support architectures. Int J Med Inform. 2008;77(10):641–9. PubMedPubMed CentralGoogle Scholar
- Wright A, Sittig DF. A framework and model for evaluating clinical decision support architectures. J Biomed Inform. 2008;41(6):982–90. PubMedPubMed CentralGoogle Scholar
- Loya SR, et al. Service oriented architecture for clinical decision support: a systematic review and future directions. J Med Syst. 2014;38(12):140. PubMedPubMed CentralGoogle Scholar
- Mandl KD, et al. The SMART platform: early experience enabling substitutable applications for electronic health records. J Am Med Inform Assoc. 2012;19(4):597–603. PubMedPubMed CentralGoogle Scholar
- Mandel JC, et al. SMART on FHIR: a standards-based, interoperable apps platform for electronic health records. J Am Med Inform Assoc. 2016;23(5):899–908. PubMedPubMed CentralGoogle Scholar
- Spineth M, Rappelsberger A, Adlassnig KP. Implementing CDS hooks communication in an Arden-syntax-based clinical decision support platform. Stud Health Technol Inform. 2018;255:165–9. PubMedGoogle Scholar
- Dolin RH, Boxwala A, Shalaby J. A pharmacogenomics clinical decision support service based on FHIR and CDS hooks. Methods Inf Med. 2018;57(S 02):e115–23. CASPubMedGoogle Scholar
- Sayeed R, Gottlieb D, Mandl KD. SMART markers: collecting patient-generated health data as a standardized property of health information technology. NPJ Digit Med. 2020;3:9. PubMedPubMed CentralGoogle Scholar
- Pelayo S, et al. Human factors and sociotechnical issues. Yearb Med Inform. 2019;28(1):78–80. PubMedPubMed CentralGoogle Scholar
- Patel VL, Kannampallil TG. Human factors and health information technology: current challenges and future directions. Yearb Med Inform. 2014;9:58–66. CASPubMedPubMed CentralGoogle Scholar
- Kushniruk A, Nohr C, Borycki E. Human factors for more usable and safer health information technology: where are we now and where do we go from here? Yearb Med Inform. 2016;1:120–5. Google Scholar
- Turner P, Kushniruk A, Nohr C. Are we there yet? Human factors knowledge and health information technology - the challenges of implementation and impact. Yearb Med Inform. 2017;26(1):84–91. CASPubMedPubMed CentralGoogle Scholar
- Carayon P, et al. Human factors systems approach to healthcare quality and patient safety. Appl Ergon. 2014;45(1):14–25. PubMedGoogle Scholar
- Carayon P, et al. Work system design for patient safety: the SEIPS model. Qual Saf Health Care. 2006;15(Suppl 1):i50–8. PubMedPubMed CentralGoogle Scholar
- Zhang J, Walji MF. TURF: toward a unified framework of EHR usability. J Biomed Inform. 2011;44(6):1056–67. PubMedGoogle Scholar
- Kushniruk AW, Patel VL. Cognitive and usability engineering methods for the evaluation of clinical information systems. J Biomed Inform. 2004;37(1):56–76. PubMedGoogle Scholar
- Borycki EM, et al. Use of qualitative methods across the software development lifecycle in health informatics. Stud Health Technol Inform. 2011;164:293–7. PubMedGoogle Scholar
- Friedman CP, Wyatt J. Evaluation methods in biomedical informatics, Health informatics, vol. xvii. 2nd ed. New York: Springer; 2006. p. 386. Google Scholar
- Ammenwerth E, Rigby M. Evidence-based health informatics: promoting safety and efficency through scientific methods and ethical policy. Studies in health technology and informatics, vol. xv. Amsterdam; Washington, DC: IOS Press; 2016. 369 pages. Google Scholar
- Cognitive informatics in health and biomedicine. Understanding and modeling health behaviors. New York, NY: Springer Berlin Heidelberg. pages cm; 2017. Google Scholar
- Eccles M, Mittman B. Welcome to implementation science. Implement Sci. 2006:1(1). Google Scholar
- Bauer MS, et al. An introduction to implementation science for the non-specialist. BMC Psychol. 2015;3:32. PubMedPubMed CentralGoogle Scholar
- Bauer MS, Kirchner J. Implementation science: what is it and why should I care? Psychiatry Res. 2020;283:112376. PubMedGoogle Scholar
- Nilsen P. Making sense of implementation theories, models and frameworks. Implement Sci. 2015;10:53. PubMedPubMed CentralGoogle Scholar
- Tabak RG, et al. Bridging research and practice: models for dissemination and implementation research. Am J Prev Med. 2012;43(3):337–50. PubMedPubMed CentralGoogle Scholar
- World Health Organization, Monitoring and evaluating digital health interventions: a practical guide to conducting research and assessment. 2016. Google Scholar
- Murray E, et al. Evaluating digital health interventions: key questions and approaches. Am J Prev Med. 2016;51(5):843–51. PubMedPubMed CentralGoogle Scholar
- Institute of Medicine (U.S.). Committee for Evaluating Medical Technologies in Clinical Use., Institute of Medicine (U.S.). Division of health sciences policy., and Institute of Medicine (U.S.). division of health promotion and disease prevention., Assessing medical technologies, vol. xvii. Washington, D.C.: National Academy Press; 1985. p. 573. Google Scholar
- Gaglio B, Shoup JA, Glasgow RE. The RE-AIM framework: a systematic review of use over time. Am J Public Health. 2013;103(6):e38–46. PubMedPubMed CentralGoogle Scholar
- Glasgow RE, et al. RE-AIM planning and evaluation framework: adapting to new science and practice with a 20-year review. Front Public Health. 2019;7:64. PubMedPubMed CentralGoogle Scholar
- Feldstein AC, Glasgow RE. A practical, robust implementation and sustainability model (PRISM) for integrating research findings into practice. Jt Comm J Qual Patient Saf. 2008;34(4):228–43. PubMedGoogle Scholar
- Damschroder LJ, et al. Fostering implementation of health services research findings into practice: a consolidated framework for advancing implementation science. Implement Sci. 2009;4:50. PubMedPubMed CentralGoogle Scholar
- Tharwat A. Classification assessment methods. Applied Computing and Informatics, 2018. Google Scholar
- Hamilton AB, Finley EP. Qualitative methods in implementation research: an introduction. Psychiatry Res. 2019;280:112516. PubMedPubMed CentralGoogle Scholar
- Anderson JG, Aydin CE. Evaluating the organizational impact of healthcare information systems. 2nd ed. health informatics series. New York, NY: Springer. xv; 2005. p. 344. Google Scholar
- Cummings E, Borycki EM. Grounded theory evolution and its application in health informatics. Stud Health Technol Inform. 2011;164:286–92. PubMedGoogle Scholar
Author information
Authors and Affiliations
- Brown University, Providence, RI, USA Elizabeth S. Chen
- Elizabeth S. Chen
You can also search for this author in PubMed Google Scholar
Corresponding author
Editor information
Editors and Affiliations
- Duke University, Durham, NC, USA Jessica D. Tenenbaum
- Six Aims for Behavioral Health, Minneapolis, MN, USA Piper A. Ranallo
Rights and permissions
Copyright information
© 2021 Springer Nature Switzerland AG
About this chapter
Cite this chapter
Chen, E.S. (2021). What Is Informatics?. In: Tenenbaum, J.D., Ranallo, P.A. (eds) Mental Health Informatics. Health Informatics. Springer, Cham. https://doi.org/10.1007/978-3-030-70558-9_2
Download citation
- DOI : https://doi.org/10.1007/978-3-030-70558-9_2
- Published : 19 November 2021
- Publisher Name : Springer, Cham
- Print ISBN : 978-3-030-70557-2
- Online ISBN : 978-3-030-70558-9
- eBook Packages : MedicineMedicine (R0)
Share this chapter
Anyone you share the following link with will be able to read this content:
Get shareable link
Sorry, a shareable link is not currently available for this article.
Copy to clipboard
Provided by the Springer Nature SharedIt content-sharing initiative